Artificial General Intelligence: Beyond Mechanistic Definitions
- David Ando Rosenstein
- Sep 10, 2024
- 3 min read
Artificial General Intelligence (AGI) is often seen as the next frontier in the development of machine intelligence, representing systems that can learn, adapt, and operate across a wide variety of contexts—unlike current AI, which is typically task-specific. AGI envisions machines capable of performing any intellectual task that a human can, with an adaptable skill set and context-aware intelligence. These systems would possess the ability to self-learn, improving their performance and expanding their knowledge base without explicit programming for each new task or domain.
What Makes AGI Different?
Unlike narrow AI, which is designed to perform specific tasks—like playing chess or analyzing data—AGI would function in a more generalized manner. It could seamlessly transition between different tasks, applying learned knowledge across various domains. For example, an AGI that excels in language processing could also learn to apply similar reasoning in scientific research or medical diagnosis without needing to be retrained from scratch. This adaptability arises from AGI’s capacity for contextual awareness, meaning it can interpret and respond to the subtleties of different situations, making it far more flexible than current AI models.
Moreover, AGI would likely operate through self-learning systems, continually refining its capabilities by interacting with the environment, much like humans do. This process involves absorbing new information, making predictions, testing hypotheses, and adjusting strategies—essentially, learning from experience. However, unlike human learning, AGI’s processing speeds would be far greater, allowing for rapid, continuous improvement across different domains.
Self-Awareness, Consciousness, and Qualia: Unanswered Questions
Despite the theoretical framework for AGI, we still face fundamental questions, particularly around concepts such as self-awareness, consciousness, and qualia (the subjective experience of perception). While AGI may mimic human cognitive functions and learn in complex, generalized ways, understanding whether a machine can ever be truly self-aware remains elusive. Can an AGI ever know that it is learning or adapting in the same way humans do, or is it simply following a set of advanced algorithms designed to mimic those behaviors?
The challenge here is avoiding overly deterministic or mechanistic definitions of these concepts. Some theories of free will, for instance, reduce human decision-making to mere biochemical reactions, which does not fully capture the richness of human experience. Similarly, mechanistic definitions of AGI could lead us to view machine intelligence as simply an upgraded form of AI, missing the deeper complexities of what true general intelligence might entail.
Functional Contextualism and AGI
Incorporating ideas from functional contextualism—particularly those found in Relational Frame Theory (RFT)—offers a more nuanced way of thinking about AGI. RFT posits that human cognition is shaped by how individuals relate and respond to various contexts. It is not just the content of what we learn but how we learn in context, based on language, social interaction, and environmental factors. This perspective could be key in developing AGI, which would need to understand not only the factual elements of a situation but also the relational and contextual aspects that influence decision-making and behavior.
For AGI to truly achieve general intelligence, it must be able to form complex relational networks, recognizing patterns not just of data but of meaning across different contexts. It would need to understand not only how to solve a math problem but why the problem matters in a given context—connecting abstract knowledge to real-world implications.
AGI's development would also need to be flexible, able to shift perspectives based on evolving environments. Rather than hardcoding rigid behaviors, functional contextualism would allow AGI to navigate the world dynamically, adapting to the relational cues and contexts it encounters. This is crucial for building a system that can not only solve predefined tasks but learn to navigate new, unpredictable situations with human-like adaptability.
Moving Forward with Caution
As we move toward the development of AGI, we must remain cautious about defining intelligence in purely mechanistic terms. While we understand certain aspects of machine learning and cognitive functions, the broader questions of consciousness, self-awareness, and qualitative experience remain. The risk of reducing AGI to a deterministic system without considering these broader elements could lead to a limited understanding of what AGI is capable of, or worse, to unforeseen consequences in its application.
By integrating functional and relational theories of cognition, we may develop AGI systems that are not only intelligent but contextually aware, capable of learning in general, human-like ways while adapting meaningfully to the world around them. However, until we fully understand the nature of consciousness and awareness, AGI remains an evolving concept—one that offers as much philosophical inquiry as technological promise.
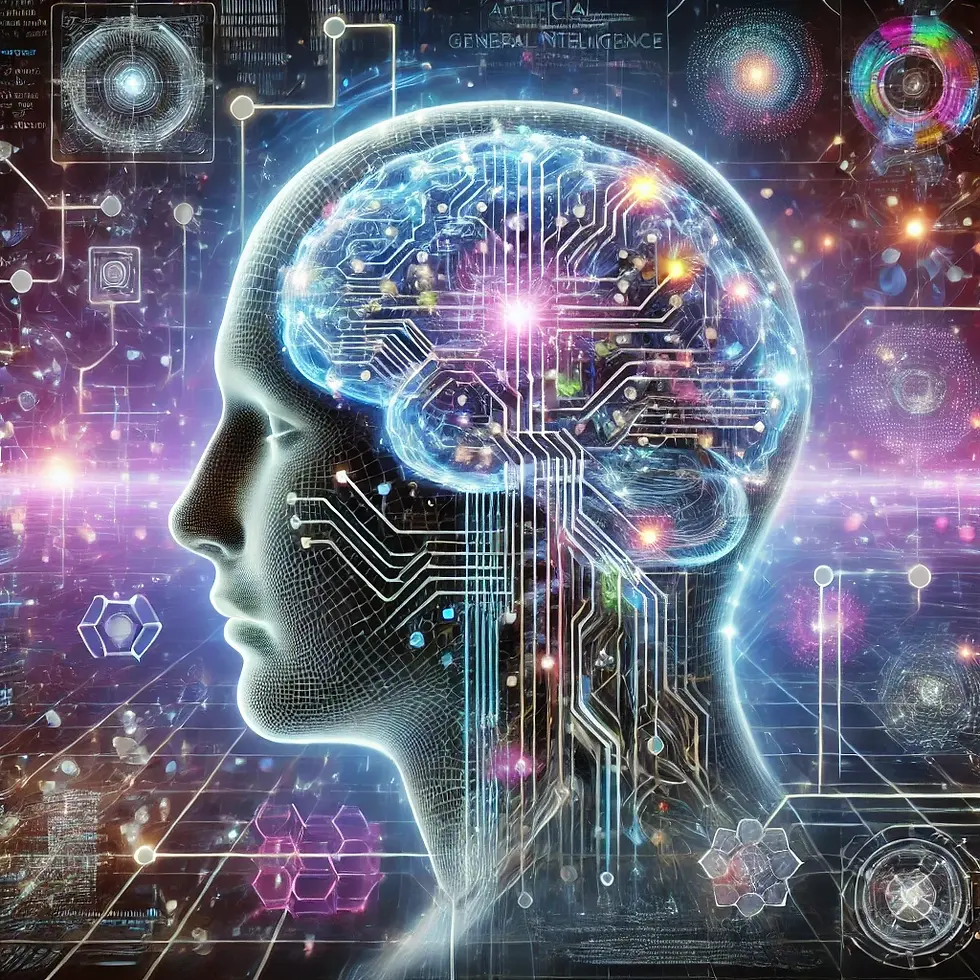
Comments